Decoding the Past Using Mice and Machines
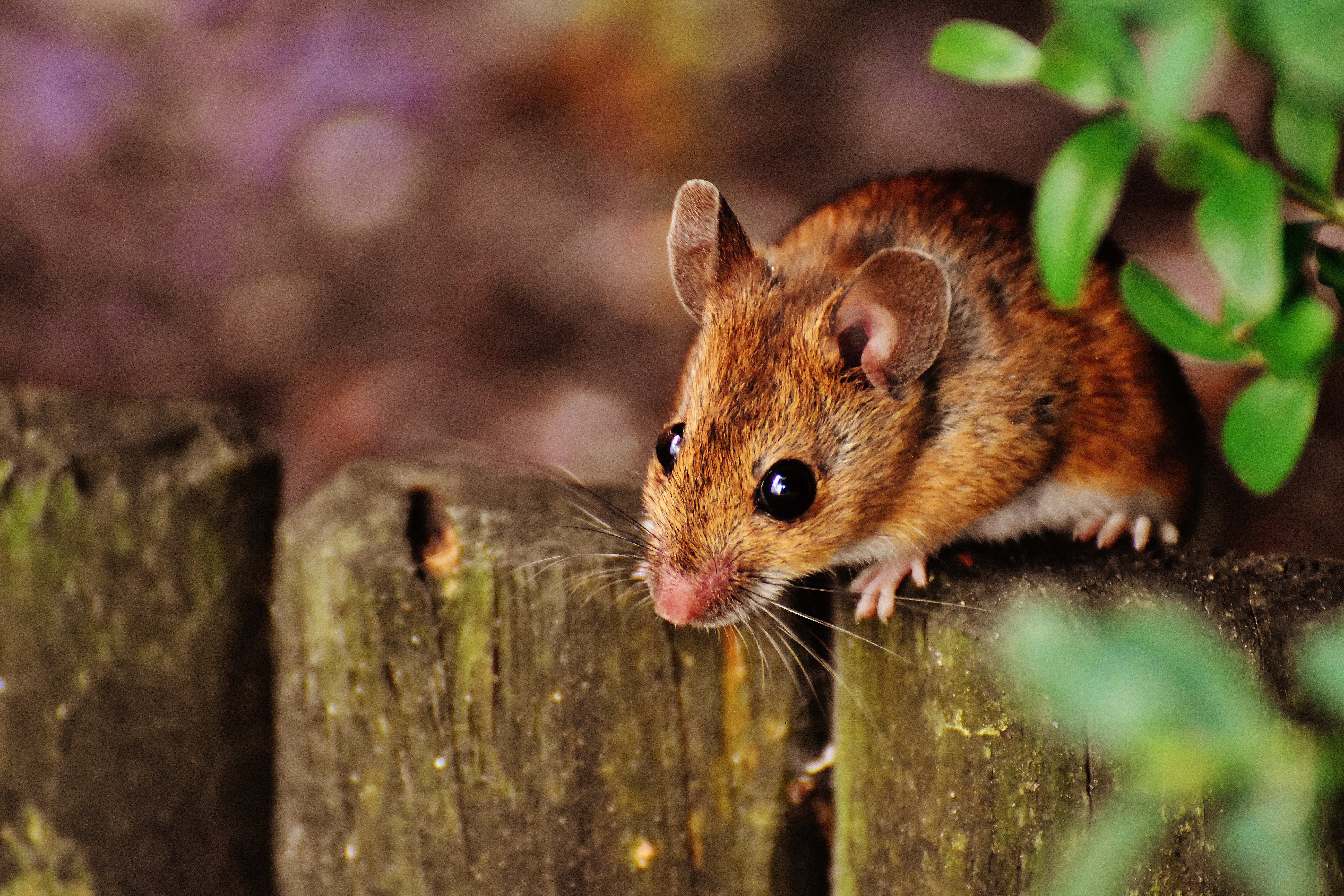
Applying Machine Learning to understand ancient climate patterns from modern rodent communities
In the quest to understand our planet’s history, scientists have traditionally relied on fossils and geological records to reconstruct past ecosystems. However, a new study, led by PhD student Pierre Linchamps and his team Emmanuelle Stoetzel, Francois Robinet, Raphaël Hanon, Pierre Latouche and Raphaël Cornette, has introduced a novel approach: using machine learning and distribution data from modern rodent communities to predict past climates in Africa. The paper Bioclimatic inference based on mammal community using machine learning regression models: perspectives for paleoecological studies published in Frontiers in Ecology and Evolution, explains that this innovative method allows for better knowledge of the structure of ancient ecosystems, which can help to resolve debates about the nature and extent of ecosystem evolution resulting from climate changes. The method also provides more detailed data collection than previous techniques, giving researchers a more comprehensive understanding of how ecosystems have changed over time, including the timing and pace of these changes.
Rodents, due to their high reproductive rates and short lifespans, respond quickly to environmental changes. This makes them excellent indicators or “proxies” for changes in their environment and they have been used successfully by other studies reconstructing other Quaternary (~ 2.6 million years ago) past environments. Dr Raphaël Hanon further explains,
“We actually started with bovids, during my PhD. As time passed, no significant progress was made regarding this side of the project. Pierre Linchamps took over for his PhD and he is a specialist on micromammals. We decided to apply the original idea to rodents, as they are really good environmental indicators. Unlike bovids, rodents are smaller and less mobile, making them diverse and sensitive to local environmental conditions. A perfect material to test our models!”
But what does machine learning mean? Although the terminology sounds daunting, the concept is straightforward. Machine learning is a subset of artificial intelligence (AI) that provides systems with the ability to automatically learn and improve from experience. Imagine you’re trying to catch fish in a river, but you don’t know what the fish look like or where they like to hang out. You start by casting your net randomly. Sometimes you catch a fish, sometimes you don’t. But as you keep doing this, you start noticing patterns – maybe you catch more fish in certain parts of the river at certain times of the day. You start adjusting your strategy based on these observations, and over time, you become more successful at catching fish. This is essentially what machine learning algorithms do: they start with a random “guess”, test how well it works, adjust based on the results, and repeat the process until they get good at the task they’re trying to accomplish. In this case, Linchamps and his team wanted to understand the relationship between the climate and where rodent species occur, using rodent distribution data and factors like temperature and rainfall.
As you feed the sets of data into your machine learning model, it starts to analyse the data and look for patterns. It might start by making random guesses, but as it processes more data, it starts to “learn” the relationships between the presence of certain mammal species and specific bioclimatic conditions. Over time, the machine learning model becomes better at predicting the bioclimatic variables based on the rodent species present in any given area. It’s adjusting its ” fishing strategy” based on the patterns it’s finding in the data.
The machine learning models of the researchers performed well, especially when predicting annual rainfall and temperature seasonality. This means that by looking at the types of rodents in an area, the models could make accurate predictions about the climate of that area. However, the team faced several challenges along the way. One of the main hurdles was the reliability of species distribution data. For example, the geographic range for widespread species is less precise than for species with restricted distributions. This could lead to an overrepresentation of widespread species in the data, which could skew the results.
Despite these challenges, the study showed that machine learning models have a strong potential for use in palaeoecology and paleoenvironmental reconstructions. This is a big deal because it provides a new tool for scientists to understand our planet’s past climates which in turn can help us predict future climate scenarios.
What Linchamps and his team have developed connects the realms of modern machine learning with ancient environments and palaeoecology. This innovative approach not only enhances our comprehension of past climates but also equips us with a powerful tool to anticipate future climate scenarios, and how they will affect our world. As we continue to grapple with the realities of climate change, such advancements highlight the importance of interdisciplinary research in shaping how we respond to our ever-changing environment.
“Our research provides a general models for African rodents. However, if you have an adequate dataset, you can apply it to any kind of environments or fauna. It also means that it reduce the results variability induced by different methods. This doesn’t mean that our method eliminates every bias, but it can at least improve the replicability of our results and the comparability between different kind of environments, ” says Dr Hanon.
This study shows us the profound potential of innovation and collaboration in science, reinforcing the notion that the keys to deciphering our future often reside not in grandiose events, but in seemingly insignificant details, such as the humble mouse.